How Top High Frequency Trading Firms Are Evolving in Today's Markets
- Bryan Downing
- 2 days ago
- 4 min read
Introduction
Over the past two decades, top high-frequency trading firms (HFT) has transformed financial markets across the globe. Characterized by ultra-fast execution, massive data processing, and algorithmic precision, HFT once stood at the frontier of trading innovation. However, as the advantages of speed diminish due to technological parity and saturated competition, HFT firms are undergoing a profound transformation. This essay explores the strategic evolution of HFT firms, focusing on their shift toward mid-frequency trading, the integration of machine learning, and their adaptation alongside hedge funds. It argues that the most successful firms are those that redefine themselves not simply as fast, but as agile, research-oriented, and data-driven entities capable of sustained innovation.
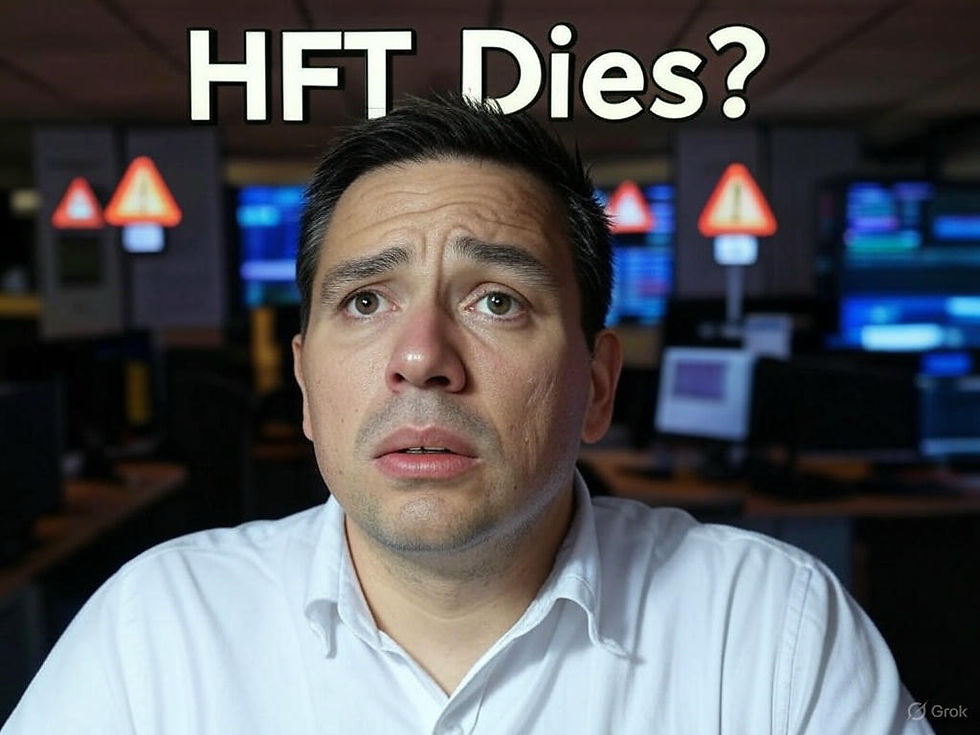
The Rise and Plateau of High-Frequency Trading
High-frequency trading emerged in the early 2000s, coinciding with the rise of electronic exchanges and the adoption of Regulation National Market System (Reg NMS) in the United States. These developments created an environment where speed became a critical asset. HFT firms invested heavily in custom hardware, co-location services, and microwave networks to minimize latency and exploit microsecond-level inefficiencies (Aldridge, 2013). By executing thousands of trades per second, they engaged in market-making, statistical arbitrage, and latency arbitrage, generating consistent, albeit slim, profit margins.
However, the initial profitability of HFT began to decline as more firms adopted similar strategies and technologies. The cost of maintaining a speed advantage escalated, while the returns on further latency reductions diminished. Market fragmentation and regulatory scrutiny further limited the effectiveness of traditional HFT strategies (Zhou & Lin, 2023). As a result, many firms recognized that success would no longer come solely from being the fastest, but from being the most adaptable.
The Strategic Shift to Mid-Frequency Trading (MFT)
As the competitive edge of pure high-frequency trading continues to erode, many leading firms are pivoting to mid-frequency trading (MFT) strategies. Unlike HFT, which emphasizes millisecond or microsecond execution, MFT operates on slightly longer timescales—ranging from hundreds of milliseconds to several minutes. This extended horizon allows traders to identify and capitalize on market inefficiencies not exclusively dependent on speed (Ding, Hanna, & Hendershott, 2014).
The shift toward MFT reflects both a tactical and operational recalibration. Firms are reallocating resources to build out MFT capabilities, recognizing that predictive modeling and behavioral analytics can offer alpha in timeframes where raw speed is less decisive. Firms like Hudson River Trading have expanded into MFT by hiring talent from multi-strategy hedge funds and diversifying their signal generation efforts (eFinancialCareers, 2024). Additionally, the slightly longer timeframes of MFT strategies allow for more complex backtesting and the incorporation of machine learning techniques, which are often computationally intensive.
Hedge Funds Entering the Arena
Hedge funds, traditionally associated with longer-horizon investing and fundamental analysis, are now integrating aspects of HFT into their operations. This convergence is driven by the pursuit of execution efficiency and short-term alpha. Firms such as Citadel and Two Sigma have developed internal HFT-style teams, often recruiting from established HFT firms to bring in expertise in low-latency execution and quantitative modeling (Chaboud et al., 2014).
While hedge funds may not match the infrastructure sophistication of pure HFT firms, their substantial capital and flexible mandates allow them to adapt quickly. However, cultural and operational differences—such as slower innovation cycles and stricter risk protocols—can pose challenges. Nevertheless, the mutual encroachment between HFT and hedge funds is dissolving traditional distinctions and fostering a more integrated trading environment.
Machine Learning: The New Battleground
Machine learning is rapidly becoming central to the next generation of trading strategies. Unlike traditional models, ML algorithms can adapt to new data, identify nonlinear relationships, and process unstructured information—from news sentiment to satellite imagery. Firms such as Jane Street and Two Sigma are at the forefront of this shift, employing large ML teams to develop models that enhance both signal accuracy and execution efficiency (Gu, Kelly, & Xiu, 2020).
The integration of machine learning also reshapes talent needs and infrastructure design. Cloud computing, GPU clusters, and scalable data pipelines are becoming as important as low-latency networks. Despite challenges around interpretability and overfitting, the use of ML enables firms to uncover patterns and opportunities that were previously inaccessible (CFA Institute, 2023).
Redefining the HFT Firm
The modern HFT firm is no longer defined solely by speed. It is characterized by strategic diversity, technological depth, and organizational agility. Modular team structures, cross-disciplinary collaboration, and flexible infrastructure allow firms to quickly pivot between strategies and adapt to market conditions. Firms like DRW, XTX Markets, and Citadel Securities exemplify this transformation, blending HFT efficiency with research-driven innovation (McKinsey & Company, 2023).
This redefinition also involves a cultural shift. Secretive, execution-focused cultures are giving way to transparent, research-oriented environments that attract talent from fields as varied as physics, computational linguistics, and applied mathematics. These changes reflect a deeper understanding that sustained success in quantitative trading requires more than raw speed—it demands continuous learning, experimentation, and strategic flexibility.
Conclusion
The evolution of high-frequency trading firms marks a significant turning point in algorithmic finance. Faced with diminishing returns from speed alone, these firms have diversified into mid-frequency strategies, integrated machine learning, and adopted more flexible organizational models. At the same time, hedge funds are adopting elements of HFT, creating a dynamic and hybridized trading landscape.
Machine learning has emerged as a particularly powerful tool, enabling firms to process complex data and generate adaptive strategies. However, its effective application requires robust infrastructure and cross-functional expertise. The firms that thrive today are those that can combine speed, intelligence, and flexibility—in essence, redefining what it means to be an HFT firm.
As the boundaries between firm types continue to blur, the future of trading will be shaped by those who can adapt swiftly, think deeply, and innovate continuously.
Comentarios