The world of Natural Language Processing (NLP) is abuzz with innovation which is smart AI. From chatbots that hold engaging conversations to seamless language translation and concise text summarization, NLP applications are transforming how we interact with information. However, one advancement poised to redefine text generation is Retrieval-Augmented Generation (RAG). This blog delves into the heart of RAG, exploring its benefits and the vast potential it holds for various applications.
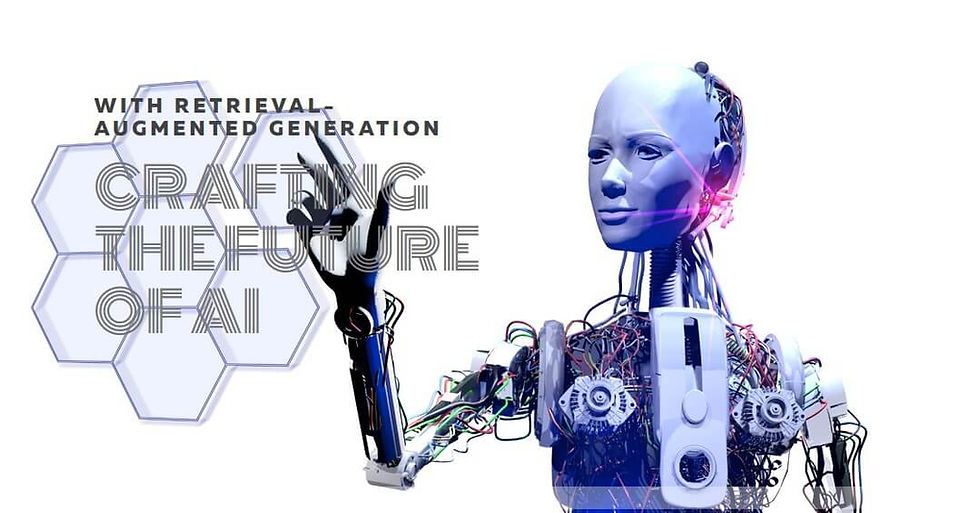
Demystifying RAG: A Symbiosis of Retrieval and Generation
RAG operates at the intersection of two powerful NLP techniques: retrieval-based models and generation-based models. Retrieval-based models excel at finding relevant information from vast datasets. Imagine a skilled librarian who can unearth the perfect book from a seemingly endless library. Similarly, RAG leverages retrieval models to identify the most pertinent information for a specific task.
Generation-based models, on the other hand, are masters of crafting text. They can generate different creative text formats, from emails to poems, based on the information they are given. Think of them as skilled writers who can transform raw data into compelling narratives.
RAG bridges the gap between these two approaches. It first utilizes the retrieval model to locate relevant information from a knowledge base, external documents, or even the internet. This retrieved information then becomes the foundation upon which the generation model builds its text. The result? Text that is not only grammatically correct and fluent but also factually accurate, informative, and highly relevant to the specific context.
Beyond Mere Fluency: The Advantages of RAG
Traditional language generation models, while impressive, often struggle with factual accuracy and context-awareness. They may generate grammatically sound sentences that lack substance or stray from the intended topic. RAG addresses these limitations by grounding the generation process in retrieved information. This leads to several key advantages:
Enhanced Factual Accuracy: By relying on external knowledge sources, RAG mitigates the risk of factual errors and ensures the generated text aligns with reality. This is particularly valuable for tasks like summarizing research papers or creating educational content.
Improved Contextual Relevance: RAG tailors the generated text to the specific context of the prompt or query. It doesn't just produce generic text; it crafts content that directly addresses the user's needs or the task at hand.
Reduced Bias: Large language models can inherit biases from the data they are trained on. RAG, by incorporating external knowledge sources, offers a potential avenue to mitigate these biases and generate more objective text.
A World of Applications: Where RAG Shines
The potential applications of RAG are vast and constantly evolving. Here are just a few examples of how this technology can be harnessed to revolutionize various fields:
Question Answering Systems: Imagine a virtual assistant that not only understands your questions but also retrieves relevant information and integrates it seamlessly into its answers. RAG can empower such systems to provide comprehensive and informative responses.
Machine Translation: Accurately translating nuances of language remains a challenge. RAG can bridge the gap by incorporating cultural context and background information retrieved from external sources, leading to more accurate and natural-sounding translations.
Content Creation: Creating engaging and informative content can be time-consuming. RAG can assist writers by generating drafts, summarizing complex information, and even suggesting relevant sources for further research.
Education and Training: Imagine personalized learning experiences where educational materials are tailored to individual student needs. RAG can generate customized lear
The Future of NLP: A Collaborative Dance Between Humans and Machines
RAG represents a significant step forward in NLP, ushering in an era of text generation that is both creative and grounded in reality. However, it is important to remember that RAG is a tool, and like any tool, its effectiveness depends on human guidance. Selecting the right knowledge sources, crafting well-defined prompts, and interpreting the generated text all require human expertise.
The future of NLP lies in a collaborative dance between humans and machines. Humans will continue to provide direction and context, while RAG and other NLP advancements will handle the heavy lifting of information retrieval and text generation. This collaborative approach holds the potential to unlock new avenues for communication, learning, and content creation, shaping a future where information is not only accessible but also tailored to our specific needs.
Comments