Rentech, Market Shocks, and the Limits of Prediction Algo
- Bryan Downing
- Apr 10
- 7 min read
Renaissance Technologies (RenTech) occupies a unique and almost mythical space in the financial world. Founded by the brilliant mathematician Jim Simons, the firm is renowned for its secretive nature of a prediction algo, its hiring of PhDs in physics, mathematics, and computer science rather than traditional finance experts, and, above all, the staggering, near-unbelievable returns of its flagship Medallion Fund. Medallion, famously closed to outside investors for decades, operates as a black box, its strategies guarded more closely than state secrets. However, RenTech also manages funds open to external capital, such as the Renaissance Institutional Equities Fund (RIEF) and the Renaissance Institutional Diversified Alpha Fund (RIDA). While these funds don't replicate Medallion's legendary performance, they still represent the pinnacle of quantitative investing and are closely watched by institutional investors and competitors alike.
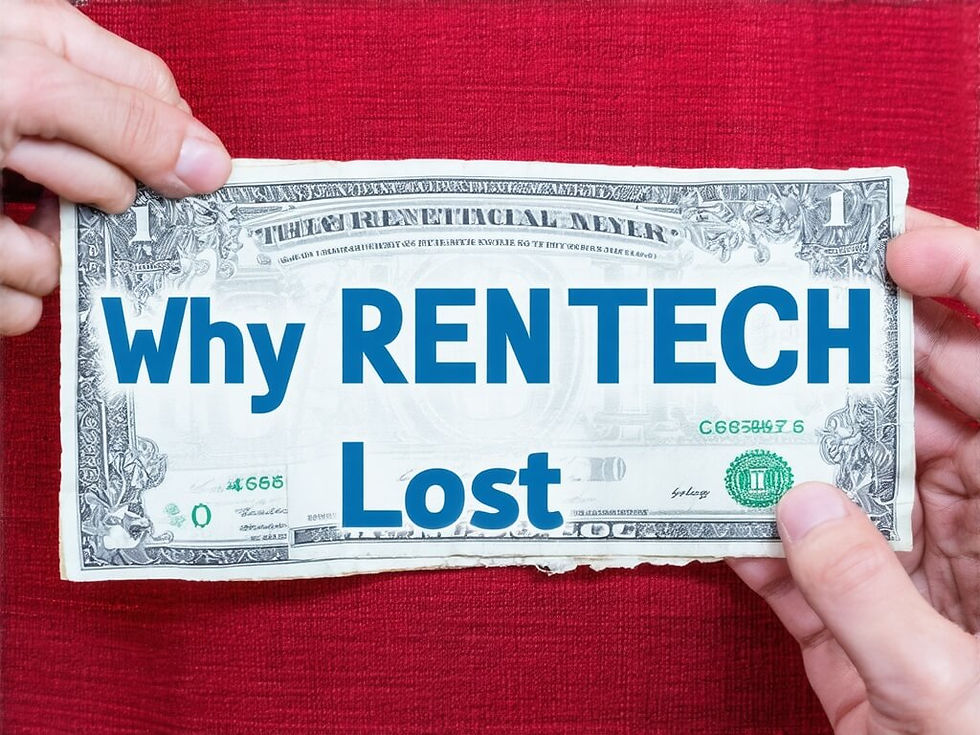
It is precisely because of this reputation for unparalleled quantitative prowess that news of significant losses sends ripples, even shockwaves, through the investment community. The report by the Financial Times detailing RIEF's sharp drawdown in April serves as a stark reminder that even the most sophisticated algorithms and powerful computing infrastructure are not immune to the sudden, often irrational, and deeply human-driven forces that can roil global markets.
The Catalyst: A Political Bolt from the Blue
The trigger for the April turmoil was reportedly a surprise announcement by former President Donald Trump regarding a potential future trade policy. His declaration of a "liberation day" policy, envisioning a sweeping 10% blanket tariff on all US imports and potentially much higher duties on specific nations, acted as a potent catalyst for market repricing.
Why would such an announcement, concerning a potential policy from a former president not currently in office, cause such immediate and violent market reactions? Several factors converge:
Uncertainty Injection: Financial markets abhor uncertainty. A blanket tariff represents a fundamental shift in global trade dynamics, potentially disrupting intricate supply chains, raising input costs for businesses, fueling consumer price inflation, inviting retaliatory tariffs from trading partners, and ultimately dampening global economic growth. The sheer scope and lack of detail inherent in such a proposal inject massive uncertainty into forecasts for corporate earnings, inflation, and interest rates.
Repricing of Risk: Models, whether human or algorithmic, incorporate assumptions about the geopolitical and policy landscape. A sudden, credible threat of significant protectionism forces an immediate repricing of risk across asset classes. Assets perceived as vulnerable to trade wars or higher inflation (like many equities, especially multinationals) are sold off, while assets perceived as havens (often cash or specific currencies) may see inflows.
Political Plausibility: While Trump was not the incumbent, his potential return to office is a scenario markets must consider. His previous tenure saw the imposition of targeted tariffs, lending credibility to the possibility of more aggressive trade actions in the future. Markets often price in probabilities, and the perceived probability of such a policy being enacted, even if not immediate, clearly shifted.
Speed of Information: In today's hyper-connected markets, news travels instantly, and algorithmic trading systems react within microseconds. There is little time for nuanced human analysis; the initial reaction is often a rapid, model-driven adjustment based on programmed responses to keywords, sentiment analysis, and correlations.
The result, as described, was a classic risk-off event, but with a particularly painful twist. Equity indices globally retreated as investors reassessed growth prospects and geopolitical risk. More unusually, government bonds, typically a safe haven during equity sell-offs, also experienced liquidation. This simultaneous decline in stocks and bonds is particularly problematic for many portfolio construction and risk management models that rely on the historically negative correlation between these two asset classes for diversification. When both fall together, diversification benefits evaporate, and overall portfolio losses are amplified. The ultimate safe haven in this instance appeared to be cash, indicating a profound desire among investors to simply reduce exposure across the board until the dust settled.
The Quant Quagmire: Why Systematic Strategies Suffered
The description of the period as "one of the most punishing stretches for systematic strategies since the pandemic" highlights specific vulnerabilities of quantitative approaches during such events:
Model Dependence on History: Quantitative models are built on historical data, identifying patterns, correlations, and statistical relationships that have held true in the past. A sudden, unprecedented political announcement like a blanket tariff proposal may represent a structural break – an event outside the historical data distribution upon which the models were trained. Algorithms may struggle to interpret or react appropriately to genuinely novel information, especially policy shocks driven by unpredictable human decisions.
Correlation Breakdown: Many quant strategies, particularly statistical arbitrage ("stat arb") and factor-based approaches, rely on stable correlations between different assets or securities. When a macro shock causes correlations to spike or break down unexpectedly (like stocks and bonds falling together), the assumptions underpinning these strategies are violated. Pairs trades diverge, factor premiums evaporate, and models generate sell signals across the board. The "cross-correlation spike" mentioned directly points to this phenomenon.
Trend Following Woes: Trend-following strategies, which aim to capitalize on established market trends, are inherently vulnerable to sharp reversals. If markets were trending upwards before the announcement, the sudden downturn would trigger stop-losses and generate sell signals, potentially locking in losses as the models exit positions rapidly.
Leverage Amplification: Quantitative hedge funds often employ significant leverage to magnify small statistical edges into meaningful returns. While leverage boosts profits in favorable conditions, it dramatically amplifies losses during adverse moves. A sharp drawdown can quickly erode capital, especially when multiple positions move against the fund simultaneously.
Margin Calls and Forced Deleveraging: The market volatility triggered significant margin calls from prime brokers – the banks that provide leverage and financing to hedge funds. As the value of collateral (the fund's assets) falls, brokers demand more cash or securities to maintain the required margin levels. This forces leveraged funds to sell assets quickly into already falling markets ("margin unwind" or "forced de-risking"). This forced selling adds further downward pressure on prices, potentially creating a vicious cycle of falling prices, more margin calls, and further forced selling, exacerbating volatility and impacting even unrelated assets as funds liquidate whatever they can. The report's mention of the largest margin calls since March 2020 underscores the severity of the pressure.
The quote, "When you see Treasuries and stocks selling off at the same time, and margin calls hitting desks globally, it’s not a great setup for leveraged quant funds," perfectly encapsulates the toxic combination of factors that made this environment so challenging for systematic, leveraged players.
RIEF vs. RIDA: A Divergence Explained
The stark difference in performance between RIEF (-8%) and RIDA (-2.4%) within the same firm is illuminating. It underscores that "quantitative investing" is not monolithic; the specific strategies, asset class exposures, time horizons, and risk parameters matter enormously.
Renaissance Institutional Equities Fund (RIEF): As its name suggests, RIEF likely has a primary focus on equities. Its models are geared towards identifying alpha (excess returns) within the stock market, potentially using statistical arbitrage, factor investing, or short-term predictive signals. In an environment where broad equity indices fell sharply and correlations spiked, a fund concentrated in equities, even one aiming for market neutrality or specific factor exposures, would likely face significant headwinds. The direct hit to its core asset class, combined with potential disruptions to its arbitrage or factor models due to the market chaos, explains the steeper drawdown. Its larger size ($19.6bn) might also imply slightly less nimbleness compared to smaller vehicles, although RenTech's execution capabilities are legendary.
Renaissance Institutional Diversified Alpha Fund (RIDA): The "Diversified Alpha" name is key. RIDA likely employs a broader range of strategies across multiple asset classes – potentially including equities, but also fixed income, currencies, commodities, and volatility. Its models might focus on different types of signals (e.g., macro trends, cross-asset relative value) that are less correlated with pure equity market movements. This diversification likely provided a crucial buffer. While some components of RIDA might have suffered, others may have held up better or even profited, leading to a much smaller overall loss. Its smaller asset base ($3.6bn) could also potentially allow for trading in slightly different, perhaps less crowded or more capacity-constrained, strategies.
This divergence highlights a critical aspect of institutional investing and fund construction: diversification across strategies and asset classes, even within a single manager's quantitative framework, can be vital for navigating turbulent periods. RIEF's performance shows the vulnerability of even sophisticated equity-focused quant strategies to macro shocks, while RIDA's relative resilience demonstrates the potential benefits of a multi-asset, multi-strategy approach.
Renewed Scrutiny and the Quant Conundrum
Events like Renaissance's April setback inevitably lead to renewed scrutiny of quantitative investing. Critics often point to such episodes as evidence that algorithms, despite their sophistication, have an "Achilles' heel": their inability to truly understand or anticipate unprecedented events, particularly those driven by human psychology, politics, or sudden policy shifts ("regime changes").
The core challenge lies in the fundamental difference between statistical patterns and causal understanding. Models excel at identifying what has happened historically and how variables have correlated. They struggle, however, with understanding the why behind those relationships and predicting how those relationships might change when underlying conditions are fundamentally altered by events outside the scope of their training data. A political decision to impose tariffs is not easily captured by analysing past price movements alone.
This doesn't invalidate quantitative investing. For decades, firms like Renaissance have proven that systematic, data-driven approaches can generate significant, consistent alpha. However, it does highlight the inherent risks and limitations:
Model Risk: Models can be wrong, overfitted to past data, or simply become obsolete as market structures evolve.
Event Risk: Unforeseen events (pandemics, wars, major policy shifts) can disrupt historical patterns and cause models to fail spectacularly.
Crowding Risk: As quantitative strategies become more popular, successful signals can become crowded, reducing their efficacy and increasing the potential for sharp, correlated unwinds when conditions change.
Execution Risk: In volatile, illiquid markets, even the best signals can be difficult to execute profitably, and forced deleveraging can exacerbate losses.
Conclusion: Humility in the Age of Algorithms
The sharp April losses experienced by Renaissance Technologies' RIEF fund, triggered by a surprise political announcement, serve as a powerful case study in the complexities and fragilities of modern financial markets. It demonstrates how quickly and violently markets can react to perceived shifts in the policy landscape, leading to unusual cross-asset correlations and significant pressure on leveraged players.
While RenTech remains an undisputed leader in quantitative finance, this episode underscores that no strategy is infallible. The reliance of quantitative models on historical data makes them inherently vulnerable to unprecedented shocks, particularly those stemming from the unpredictable realm of politics. The divergence between RIEF and RIDA further highlights the importance of strategy design and diversification, even within a sophisticated quantitative framework.
Ultimately, the event is a lesson in humility. It reminds investors and fund managers that despite the immense power of data analysis and algorithmic trading, markets remain complex systems influenced by a multitude of factors, including human behaviour, political decisions, and unforeseen global events. Navigating these waters requires not only sophisticated models but also robust risk management, an awareness of the limitations of any predictive system, and perhaps a degree of caution regarding the potential for the unexpected to reshape the investment landscape in the blink of an eye. The quant king may have stumbled, but the lessons learned from that stumble resonate across the entire kingdom of finance.
Comments