How useful is probability and statistics in finance for success
- Bryan Downing
- 12 minutes ago
- 8 min read
The Quantitative Compass: How Probability and Statistics Navigate the World of Finance
Finance, at its heart, is the science of managing money, encompassing activities like investing, borrowing, lending, budgeting, saving, and forecasting. This involved stats in finance. Underlying all these activities is a fundamental, unavoidable element: uncertainty. How useful is probability and statistics in finance for success? Future market movements, interest rates, borrower creditworthiness, the occurrence of insured events – none of these can be predicted with absolute certainty. In this landscape of unknowns, probability and statistics emerge not just as helpful tools, but as the indispensable quantitative compass guiding decision-making, risk assessment, and value creation.
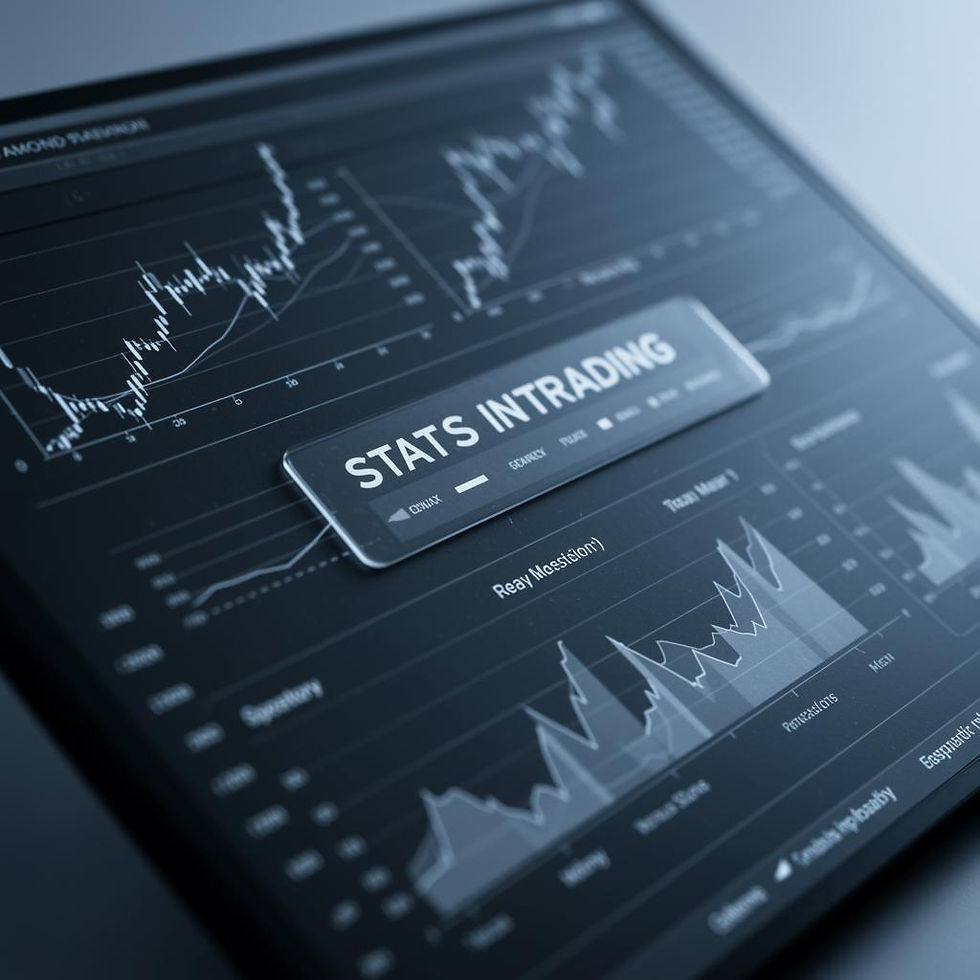
From the intricate pricing of exotic derivatives to the foundational principles of portfolio diversification, the fingerprints of probability theory and statistical analysis are everywhere in modern finance. They provide the framework for quantifying uncertainty, identifying patterns, testing hypotheses, and building models that attempt to bring order to the apparent chaos of financial markets. This article explores the multifaceted ways in which these mathematical disciplines underpin virtually every aspect of the financial world.
1. Quantifying and Managing Risk: The Bedrock Application
Perhaps the most crucial role of probability and statistics in finance is in the measurement and management of risk. Risk, in financial terms, is the possibility that an actual return will differ from an expected return, often implying the potential for loss.
Probability Distributions: Understanding the likelihood of different outcomes is paramount. Asset returns are often modeled using probability distributions. While the normal distribution (the "bell curve") is a common starting point due to its simplicity and the Central Limit Theorem, financial professionals recognize its limitations, particularly its failure to capture "fat tails" – the higher-than-expected probability of extreme market events. Consequently, other distributions like Student's t-distribution or skewed distributions are often employed to better model the observed behavior of financial asset returns. Probability allows analysts to ask: "What is the chance that this stock will lose more than 10% of its value next month?"
Volatility (Standard Deviation): The most widely used measure of risk for an asset is its volatility, typically calculated as the standard deviation of its returns. Standard deviation, a core statistical concept, measures the dispersion or spread of data points (returns) around their average (mean return). Higher standard deviation implies greater uncertainty and risk.
Value at Risk (VaR): A cornerstone of modern risk management, VaR is a statistical measure estimating the potential loss in value of an asset or portfolio over a defined period for a given confidence interval. For example, a 1-day 95% VaR of $1 million means there is a 5% probability that the portfolio will lose more than $1 million over the next trading day, assuming normal market conditions. Calculating VaR relies heavily on understanding the probability distribution of portfolio returns.
Conditional VaR (Expected Shortfall): Recognizing that VaR only tells you the threshold of loss, not how much you could lose if that threshold is breached, CVaR (or Expected Shortfall) was developed. It calculates the expected loss given that the loss exceeds the VaR level, providing a better measure of tail risk.
Monte Carlo Simulation: When analytical solutions are complex or impossible (e.g., pricing complex options or modeling portfolio risk with many interacting assets), Monte Carlo methods are used. These involve running thousands or millions of simulated random trials based on probabilistic assumptions about underlying variables (stock prices, interest rates) to generate a distribution of possible outcomes. This statistical technique allows for the analysis of complex scenarios and the estimation of probabilities for various financial results.
Stress Testing and Scenario Analysis: Beyond standard statistical measures, banks and financial institutions use stress testing. This involves statistically modeling the impact of extreme, but plausible, adverse scenarios (e.g., a severe recession, a sharp interest rate hike, a market crash) on their portfolios and capital adequacy. Probability helps define the severity and likelihood of these scenarios.
2. Portfolio Management and Investment Theory
Modern Portfolio Theory (MPT), pioneered by Harry Markowitz (who won a Nobel Prize for his work), is fundamentally built on statistical concepts.
Expected Return and Variance: MPT assumes investors are risk-averse and aim to maximize expected return for a given level of risk (variance/standard deviation) or minimize risk for a given level of expected return. Calculating these expected values and variances relies on historical data analysis and probabilistic forecasting.
Correlation and Covariance: The magic of diversification stems from these statistical measures. Correlation quantifies the degree to which two assets' returns move together. By combining assets with low or negative correlations, the overall portfolio volatility can be reduced without necessarily sacrificing expected return. Covariance, a related measure, is used directly in the calculation of portfolio variance. Statistics provides the tools to measure these relationships.
The Efficient Frontier: MPT uses statistical optimization techniques to identify the set of portfolios offering the highest expected return for each level of risk. This optimal set forms the "Efficient Frontier." Investors choose portfolios on this frontier based on their individual risk tolerance.
Capital Asset Pricing Model (CAPM): This cornerstone model uses statistical regression to relate the expected return of an asset to its systematic risk (risk that cannot be diversified away), measured by Beta (β). Beta is calculated by regressing the asset's returns against the market's returns. A Beta greater than 1 suggests the asset is more volatile than the market, while less than 1 suggests lower volatility. CAPM relies heavily on concepts like expected value, variance, covariance, and linear regression.
Performance Measurement (Sharpe Ratio, Sortino Ratio): Evaluating investment performance requires statistical metrics. The Sharpe Ratio measures risk-adjusted return by calculating the excess return (portfolio return minus risk-free rate) per unit of total risk (standard deviation). The Sortino Ratio is similar but only considers downside deviation (volatility of negative returns), which some argue is a more relevant measure of risk for investors.
3. Pricing Derivatives and Financial Engineering
The pricing of options, futures, swaps, and other derivatives is deeply rooted in probability and stochastic calculus (the mathematics of random processes).
Black-Scholes-Merton Model: This Nobel Prize-winning model for pricing European options is a prime example. While its derivation involves complex stochastic differential equations, its core relies on assumptions about the probability distribution of the underlying asset's price (typically assumed to follow a geometric Brownian motion, leading to a lognormal distribution of prices) and the concept of risk-neutral pricing, which uses probabilities adjusted for risk.
Binomial Option Pricing Model: A simpler model that breaks down the time to expiration into discrete steps. At each step, the underlying asset price is assumed to have a certain probability of moving up or down by a specific amount. By working backward from expiration, the model calculates the option price at the present time using expected values based on these probabilities.
Risk-Neutral Probabilities: A key concept in derivative pricing is the use of "risk-neutral" probabilities. These are adjusted probabilities that allow practitioners to price derivatives by calculating expected future payoffs and discounting them back to the present using the risk-free interest rate, simplifying the complex reality of risk aversion.
4. Algorithmic and Quantitative Trading
The rise of algorithmic trading, where computers execute trades based on pre-programmed instructions, relies extensively on statistical analysis.
Signal Generation: Trading algorithms often generate buy/sell signals based on statistical patterns or indicators. This could involve identifying mean reversion opportunities (prices deviating significantly from their statistical average), momentum signals (prices trending strongly in one direction), or statistical arbitrage (exploiting tiny, temporary price discrepancies between related assets, identified through statistical analysis like pairs trading cointegration tests).
Backtesting: Before deploying a trading strategy with real capital, quantitative traders rigorously backtest it on historical data. This involves simulating the strategy's performance over past periods and analyzing statistical metrics like total return, maximum drawdown (peak-to-trough decline), Sharpe ratio, win rate, and profit factor. Statistical significance testing (e.g., using p-values) helps determine if the observed performance is likely genuine or just due to random chance (data snooping).
Machine Learning Models: Increasingly, AI and machine learning (which are heavily based on statistics) are used to develop trading strategies. Techniques like regression, classification (e.g., predicting market direction), clustering (identifying market regimes), and reinforcement learning are employed to analyze vast datasets and uncover complex trading patterns.
5. Insurance and Actuarial Science
The entire insurance industry is built upon probability and statistics. Actuaries are specialized professionals who use these tools extensively.
Pricing Premiums: Insurance premiums are calculated based on the probability of the insured event occurring (e.g., death, car accident, property damage) and the expected cost (severity) if it does occur. Actuaries use historical data and statistical models (like mortality tables in life insurance) to estimate these probabilities and expected values.
Law of Large Numbers: A fundamental statistical principle stating that as the number of policyholders increases, the actual loss per policyholder will converge towards the expected loss. This allows insurance companies to pool risk effectively and operate profitably despite the uncertainty of individual claims.
Reserving: Insurers must hold adequate reserves to pay future claims. Statistical forecasting techniques are used to estimate the amount needed based on projected claim frequencies and severities.
6. Credit Risk Modeling
Lenders (banks, credit card companies) use statistical models to assess the risk that borrowers will default on their obligations.
Probability of Default (PD): Statistical models (often using techniques like logistic regression or machine learning classifiers) are built using historical borrower data (income, credit history, debt levels, etc.) to estimate the probability that a specific borrower will default within a certain timeframe.
Loss Given Default (LGD): This estimates the proportion of the exposure that will be lost if a borrower defaults. Statistical analysis of historical recovery rates on defaulted loans informs LGD estimates.
Exposure at Default (EAD): This estimates the total amount outstanding if a borrower defaults (e.g., for a credit card, this considers the possibility of the borrower drawing down their remaining credit line before defaulting).
Credit Scoring: Consumer credit scores (like FICO) are themselves statistical models that condense various data points into a single number predicting creditworthiness.
7. Market Analysis and Economic Forecasting
Statistics provides the tools for analyzing market trends, testing economic theories, and building forecasts.
Descriptive Statistics: Summarizing market data (e.g., average market return, range of price movements) provides a basic understanding.
Inferential Statistics: Drawing conclusions about a larger market based on a sample of data (e.g., testing if a particular trading strategy yields statistically significant positive returns).
Regression Analysis: Identifying relationships between variables (e.g., how changes in interest rates affect stock market returns, or how advertising spend impacts company sales).
Time Series Analysis: Analyzing data points collected over time to identify trends, seasonality, and cyclical patterns, and to make forecasts (e.g., using models like ARIMA or GARCH to forecast stock prices or volatility).
Limitations and Caveats
Despite their immense power, probability and statistics are not crystal balls. Their application in finance faces challenges:
Assumptions: Many models rely on simplifying assumptions (e.g., normal distribution of returns, constant volatility, market efficiency) that may not hold true in reality, especially during crises.
Non-Stationarity: Financial markets evolve; relationships and statistical properties (like volatility or correlations) can change over time, rendering models trained on past data less effective.
Data Quality: Models are only as good as the data they are fed. Inaccurate, incomplete, or biased data leads to flawed conclusions.
Black Swan Events: Highly improbable, unpredictable events with massive consequences (popularized by Nassim Taleb) are inherently difficult to capture in standard statistical models based on historical data.
Model Risk: The risk that a model is incorrectly specified or implemented, leading to poor decisions.
Conclusion: The Indispensable Language of Modern Finance
From managing the intricate risks of a global investment bank's portfolio to pricing an individual insurance policy, probability and statistics provide the essential framework and tools for navigating the inherent uncertainties of finance. They allow practitioners to move beyond gut feeling and intuition towards rigorous, data-driven decision-making. They enable the quantification of risk, the optimization of investments, the fair pricing of complex instruments, and the testing of economic and trading theories.
While acknowledging their limitations and the ever-present possibility of unforeseen events, the ability to think probabilistically and apply statistical reasoning is no longer just an advantage in finance – it is a fundamental necessity. As markets become more complex, data becomes more abundant, and computational power increases, the role of probability and statistics in decoding financial behavior, managing risk, and creating value will only continue to grow in importance. They are, truly, the quantitative compass by which the modern financial world orients itself.
Comments